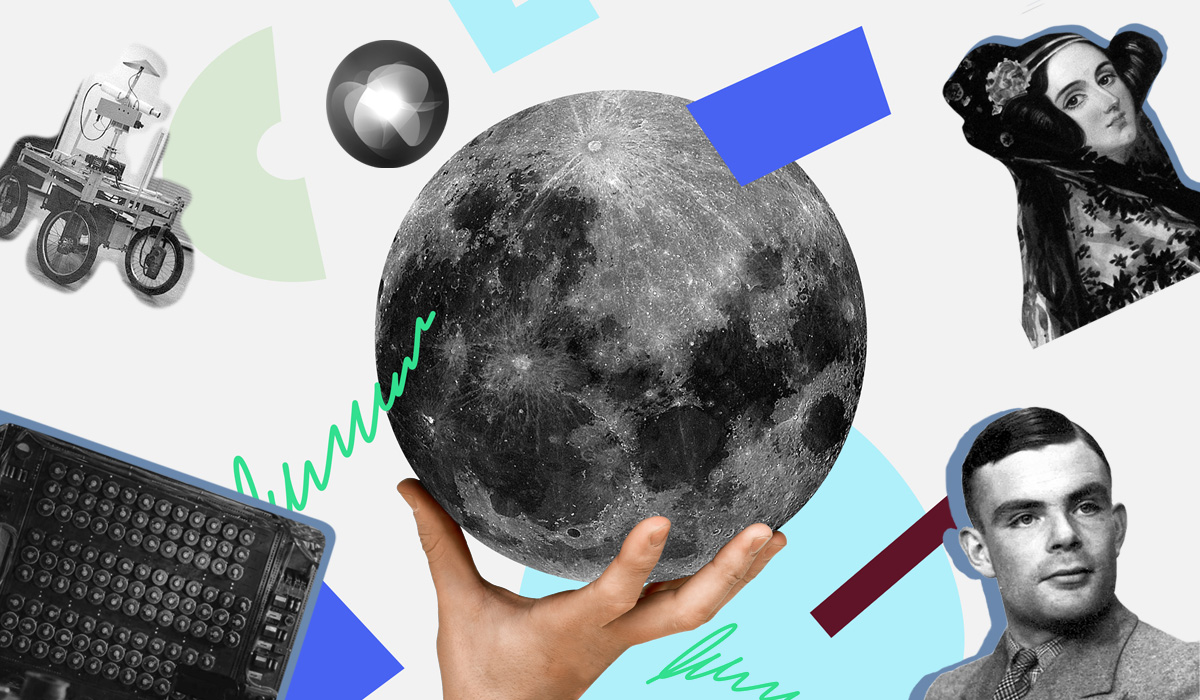
The history of Artificial Intelligence (AI) is also the history of Machine Learning (ML) and Deep Learning (DL). When talking about AI we also must talk about how its subfields, ML and DL, developed simultaneously and, little by little, amplified their field of expertise.
The history of Artificial Intelligence is not entirely linear. Throughout the years, there have been significant discoveries, but also the so-called "AI winters."
This article will briefly cover the most outstanding events of the prehistory, history, and revolution of Artificial Intelligence, as well as the beginning and development of Machine Learning and Deep Learning.
Table of Contents
- The Prehistory of Artificial Intelligence: 1700 - 1900
- The Beginning of the Computational Era: 1900 - 1950
- The Beginning of Artificial Intelligence 1950 - 2000
- The Evolution of Artificial Intelligence: 2000 - 2023
- The History of Machine Learning
- The History of Deep Learning
The Prehistory of Artificial Intelligence: 1700 - 1900
3 CRUCIAL CHARACTERS IN THE PREHISTORY OF ARTIFICIAL INTELLIGENCE 1600 - 1850
1623: Wilhelm Schickard creates the calculator
Wilhelm Schickard was a prominent German teacher, mathematician, theologian, and cartographer. Wilhelm invented several machines for various purposes, but his most notable contributions were the first mechanical calculator and a machine for learning Hebrew grammar.
In 1623, Wilhelm Schickard invented a device that allowed him to perform arithmetic operations completely mechanically, he called it the calculating clock.
Its operation was based on rods and gears that mechanized the functions that were previously performed manually.
1822: Charles Babbage built the mechanical calculator.
He is considered the father of modern printers and a pioneer of computing.
In 1822, Babbage was able to develop and partially design a mechanical calculator capable of performing calculations in tables of numerical functions by the method of differences and designing the analytical machine to run tabulation or computation programs. Among his inventions, there is also the differential machine.
Later, Babbage worked with Ada Lovelace to translate her writing into Italian on the analytical machine. Their relationship would help to cement the principles of what would become artificial intelligence.
1830: Ada Lovelace, the first programmer
British mathematician Ada Lovelace developed contributions that still greatly impact today, such as the development of the first algorithm together with Charles Babbage.
Another important contribution of Lovelace was the concept of the universal machine. He created a device that, in theory, could be programmed and reprogrammed to perform a variety of tasks not limited to mathematical calculation, such as processing symbols, words and even music.
The Beginning of the Computational Era: 1900 - 1950
3 EVENTS THAT MARKED THE BEGINNING OF THE COMPUTATIONAL ERA 1900 - 1950
1924: Creation of IBM
Founded in 1911, it was initially a company that developed punch card counting machines. Due to its success and to reflect the organization's international growth, the company's name was changed to International Business Machines Corp. or IBM in 1924.
Their beginnings in the business would lead them to be leaders in software solutions, hardware, and services that have marked the technological advancement of this era.
The company has managed to adapt to the technological changes in the market to create innovative solutions over the years.
1936: Turing Machine
The Turing Machine was created in 1936 by Alan Turing, known as the father of Artificial Intelligence.
He created a computational model capable of storing and processing information virtually, marking the history of computing, and considered the origin of computers, cell phones, tablets, and other current technologies.
This computational model can be adapted to simulate the logic of any algorithm. Its creation demonstrated that some of these Turing machines could perform any mathematical computation if it were representable by an algorithm.
1943: First functional digital computer ENIAC
The Electronic Numerical Integrator And Computer project, ENIAC, was created in 1943 by Americans John William Mauchly and John Presper Eckert. It was conceived for military purposes but was not completed until 1945 and was presented to the public in 1946 and used for scientific research.
The machine weighed 27 tons, measured 167 square meters and consisted of 17,468 tubes. It was programmable to perform any numerical calculation, had no operating system or stored programs, and only kept the numbers used in its operations.
The Beginning of Artificial Intelligence 1950 - 2000
1950: Turing Test
Alan Turing developed the Turing test. This test aims to determine whether Artificial Intelligence can imitate human responses.
It is a conversation between a human, a computer, and another person, but without knowing which of the two conversationalists is a machine. The person asks questions to the chatbot and another person, and in case of not distinguishing the human from the machine, the computer will have successfully passed the Turing test.
1956: First Dartmouth College Conference on Artificial Intelligence
In the summer of 1956, Marvin Minsky, John McCarthy, and Claude Shanon organized the first conference on Artificial Intelligence at Dartmouth College.
This important event was the starting point of Artificial Intelligence. McCarthy coined the term Artificial Intelligence for the first time during this event. It was also determined that in the next 25 years computers would do all the work humans did at that time. In addition, theoretical logic was considered the first Artificial Intelligence program to solve heuristic search problems.
1970-1980: Expert systems
These systems were very popular in the 1970s. They used expert knowledge to create a program in which a user asks a question to the system to receive an answer and it is categorized as useful or not.
The software uses a simple design and is reasonably easy to design, build and modify. These simple programs became quite useful and helped companies save large amounts of money. Today, these systems are still available but their popularity has declined over the years.
1974-1980: First AI winter
The term "AI winter" relates to the decline in interest, research, and investment in this field.
It started when AI researchers had two basic limitations: low memory and processing speed, which is minimal compared to the technology in this decade.
This period began after the first attempts to create machine translation systems, which were used in the Cold War and ended with the introduction of expert systems that were adapted by hundreds of organizations around the world.
1980: Natural language processors
These technologies make it possible for computers and machines to understand human language.
They began to be designed to translate Russian into English for Americans in the early 1960s. Still, they did not have the expected result until 1980, when different algorithms and computational technologies were applied to provide a better experience.
1987-1993: Second AI winter
Carnegie Mellon University developed the first commercial AI system called XCON. The LISP programming language was created and became the common denominator among AI developers. Hundreds of companies invested in this system as it promised millions in profits to those who implemented it.
But in 1987, the market collapsed with the dawn of the PC era as this technology overshadowed the expensive LISP machines. Now Apple and IBM devices could perform more actions than their predecessors, making them the best choice in the industry.
1990: Intelligent Agents
Also known as bots or virtual digital assistants.
The creation and research of these systems began in 1990. They are able to interpret and process the information they receive from their environment and act based on the data they collect and analyze, to be used in news services, website navigation, online shopping and more.
The Evolution of Artificial Intelligence: 2000 - 2023
6 INVENTIONS THAT REVOLUTIONIZED AI
2011: Virtual assistants
A virtual assistant is a kind of software agent that offers services that help automate and perform tasks.
The most popular virtual assistant is undoubtedly Siri, created by Apple in 2011. Starting with the iPhone 4s, this technology was integrated into the devices. It understood what you said and responded with an action to help you, whether it was searching for something on the internet, setting an alarm, a reminder or even telling you the weather.
2016: Sophia
Sophia was created in 2016 by David Hanson. This android can hold simple conversations like virtual assistants, but unlike them, Sophia makes gestures like people and generates knowledge every time it interacts with a person, subsequently mimicking their actions.
2018: BERT by Google
BERT, designed by Google in 2018, is a Machine Learning technique applied to natural language processors, aiming to understand better the language we use every day. It analyzes all the words used in a search to understand the entire context and yield favorable user results.
It is a system that uses transformers, a neural network architecture that analyzes all possible relationships between words within a sentence.
2020: Autonomous AI
The North American firm, Algotive, develops Autonomous Artificial Intelligence algorithms that enhance video surveillance systems in critical industries.
Its algorithms rely on Machine Learning, the Internet of Things (IoT), and unique video analytics algorithms to perform specific actions depending on the situation and the organization's requirements.
vehicleDRX, its solution for public safety, is an example of how the organization's algorithms make video surveillance cameras intelligent to respond to emergencies and help officers do their jobs better.
If you are interested in learning more about Algotive's Autonomous Artificial Intelligence solutions, visit: www.algotive.ai/vehicledrx
2022: GATO by Deep Mind
The new AI system created by Deep Mind has the ability to complete more than 600 different tasks simultaneously, from writing image descriptions to controlling a robotic arm.
It acts as a vision and language model that has been trained to execute different tasks with different modalities and be performed successfully. It is expected that this system will have a larger number of actions to perform in the future and will pave the way for Artificial General Intelligence.
2022: vehicleDRX by Algotive
Algotive's novel software, vehicleDRX has the ability to identify and monitor vehicles of interest and suspicious behavior on motorcycles in real time, analyzing risky situations on the street and leveraging the video surveillance infrastructure of state governments and law enforcement agencies.
vehicleDRX enhances public safety and collaborates with command center monitors and public safety agencies. Its algorithms allow it to perform in-depth analysis, track vehicle speed and direction, perform permanent surveillance, search for vehicles and motorcycles by characteristics, create case files, and is compatible with all IP cameras.
If you are interested in knowing more about the problems that vehicleDRX solves, please visit: www.algotive.ai/vehicledrx
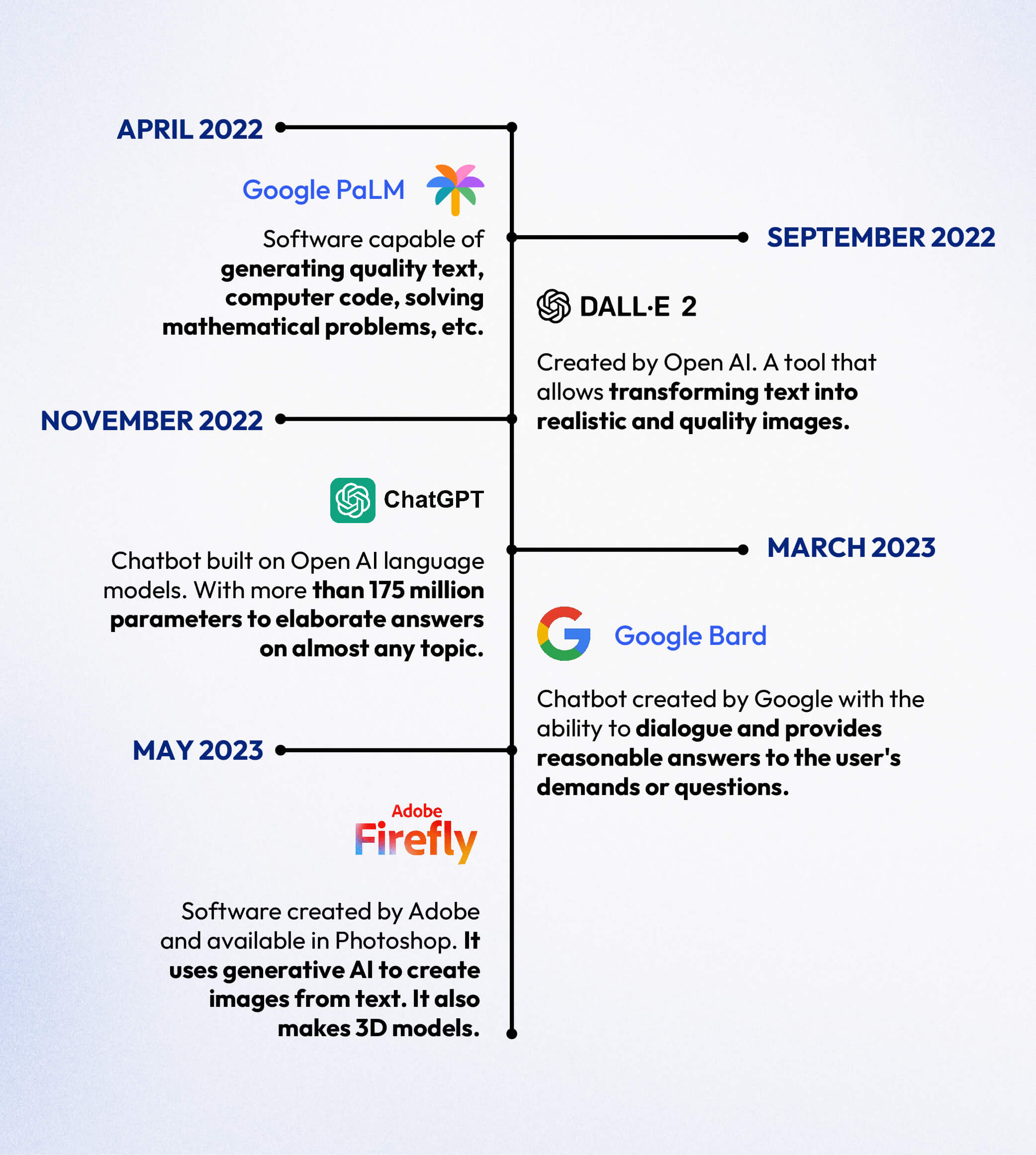
April 2022
In the early months of 2022, Google launched its AI called PaLM, or Pathways Language Model. This software can generate high-quality texts, creating computer code, solving complex math problems, and even explaining jokes with efficiency and accuracy.
In their official blog post: https://ai.googleblog.com/2022/04/pathways-language-model-palm-scaling-to.html, Google demonstrates how PaLM can do all this and other things, for example, to guess the title of a film from only 3 emojis.
PaLM works by gathering information from 540 million parameters and it was trained using data in several languages and from several sources like high-quality documents, books, real conversations, and GitHube code.
PaLM opened the path for one of Google’s most recent developments in AI: “Bard”, but first we must address other AIs that have become popular and even polemic in the last year.
September 2022
DALL-E 2 was created by Open AI as an updated version of DALL-E, launched in January 2021. This second version became widely popular because of its simple interface but complex results. This tool allows the user to create realistic, high-definition images by simply inputting text in the DALL-E 2 interface.
To have images as accurate as possible the user must input detailed descriptions and the program will generate the art taking that information into account.
This tool has been very popular in the past months as it has allowed all kinds of people to create images of very different kinds and it has allowed those with no ability to draw or paint to express themselves through images.
November 2022
Another popular and recent development in AI, ChatGPT is an AI chatbot built on top of Open AI language models, like GPT-4 and its predecessors. Through an interface that resembles a chat page, GPT is capable of engaging in conversation with the user.
Not only this, but his answers seem lively as it can “admit its mistakes, challenge incorrect premises, and reject inappropriate requests,” according to its official website (https://openai.com/blog/chatgpt).
This AI was trained first using Reinforcement Learning from Human Feedback (RLHF), with a human trainer providing conversations in which they acted as both sides: the computer and the human interviewer. ChatGPT has more than 175 million parameters from which it elaborates its answers and is able to talk about almost any subject. People all around the world have been using it to see the reach of its capabilities.
March 2023
In response to ChatGPT, Google launched its own AI chatbot, naming it Bard. It is based on LaMDA (Language Model for Dialogue Application), a model created by Google. Bard can dialogue with their interlocutors, and according to the company, it can be used as a creative and helpful collaborator, as it can help the user organize and create new ideas that can be used in several environments, from the artistic to the corporate side. This AI constantly learns as it picks up patterns from trillions of words that help predict a reasonable response to the user's questions or demands.
May 2023
One of the latest trends in popular AI, Firefly, is a software created by Adobe. Similarly, to DALL-E 2, Firefly uses Generative AI to create images from text, recolor images, created 3D models, or extend images beyond their borders by filling blank spaces. While it can be used alone, Firefly has also been introduced to the famous image-editing software, Photoshop. By using textual input, Firefly can add content to an image, delete elements, and replace entire parts of a photo, among other things.
The History of Machine Learning
MOST IMPORTANT MOMENTS IN THE HISTORY OF MACHINE LEARNING
1952: Arthur Samuel creates the first program to play checkers
Arthur Samuel is one of the pioneers of computer games and Artificial Intelligence.
In 1952 he began writing the first computer program based on Machine Learning in which he was able to give an early demonstration of the fundamental concepts of Artificial Intelligence. The software was a program that played Chinese checkers and could improve its game with each game. It was able to compete with middle-level players. Samuel continued to refine the program until it was able to compete with high-level players.
1957: Frank Rosenblatt designed the Perceptron
Perceptron is an instrument developed by psychologist Frank Rosenblatt to classify, explain and model pattern recognition skills in images.
It was the first computer built specifically to create neural networks. The Perceptron was implemented in one of IBM's computers. Thanks to it, it was able to execute 40,000 instructions per second.
1963: Donald Michie built MENACE
MENACE was a mechanical computer made of 304 matchboxes designed and built by Michie since he did not have a computer.
Michie built one of the first programs with the ability to learn to play Tic-Tac-Toe. He named it the Motor Educable Machine of Zeros and Crosses (MENACE).
The machine learned to play more and more games where it eliminated a losing strategy by the human player at every move.
1967: Nearest Neighbor Algorithm
Also known as k-NN, it is one of the most basic and essential classification algorithms in Machine Learning.
It is a supervised learning classifier that uses proximity to recognize patterns, data mining, and intrusion detection to an individual data point to classify the interest of the surrounding data.
It solves various problems such as recommender systems, semantic search, and anomaly detection.
1970: Seppo Linnainmaa and automatic differentiation
Linnainmaa published the inverse model of automatic differentiation in 1970. This method later became known as backpropagation and is used to train artificial neural networks.
Backpropagation is a set of techniques for evaluating the derivative of a function specified by a computer in which a sequence of elementary arithmetic operations (addition, subtraction, division, etc.) and elementary functions (exp, sin, log, cos, etc.) are executed to apply the chain rule by performing automatic calculations.
1979: Hans Motavec created the first autonomous vehicle
Motavec built the Standford Cart in 1979. It consisted of 2 wheels and a mobile television camera from side to side, without the need to move it.
The Standford Cart was the first autonomous vehicle controlled by a computer and capable of avoiding obstacles in a controlled environment. In that year, the vehicle successfully crossed a room full of chairs without the need for human intervention in 5 hours.
1981: Gerald Dejong and the EBL concept
Dejong introduced the "Explanation based learning" (EBL) concept in 1981, a Machine Learning method that makes generalizations or forms concepts from training examples that allow it to discard less important data or data that does not affect the investigation.
It is linked with coding to help with supervised learning.
1985: Terry Sejnowski invents NETtalk
NETtalk is an artificial neural network created by Terry Sejnowski in 1986. This software learns to pronounce words in the same way a child would. NETtalk's goal was to build simplified models of the complexity of learning cognitive tasks at the human level.
This program learns to pronounce written English text by matching phonetic transcriptions for comparison.
1990: Kearns and Valiant proposed Boosting
It is a Machine Learning meta-algorithm that reduces bias and variance in supervised learning to convert a set of weak classifiers to a robust classifier.
It combines many models obtained by a method with low predictive capability to boost it.
The idea of Valiant and Kearns was not satisfactorily solved until Freund and Schapire in 1996, presented the AdaBoost algorithm, which was a success.
1997: Jürgen Schmidhuber and Sepp Hochreiter created Speech Recognition
It is part of Deep Learning with a technique called LSTM that uses neural network models where it can learn previously done tasks.
It can collect data such as images, words, and sounds where algorithms interpret it and store this information to perform actions.
It is a technique that, with its evolution, we have come to use daily in applications and devices such as Amazon's Alexa, Apple's Siri, Google Translate, and more.
2002: Launch of Torch
Torch was an open-source library that provided an environment for numerical development, Machine Learning, and Computer Vision with a particular emphasis on Deep Learning.
It was one of the fastest and most flexible frameworks for Machine and Deep Learning, which was implemented by companies such as Facebook, Google, Twitter, NVIDIA, Intel and more.
It was discontinued in 2017 but is still used for finished projects and even developments through PyTorch.
2006: Facial recognition
Facial recognition was evaluated through 3D facial analysis and high-resolution images.
Several experiments were carried out to recognize individuals and identify their expressions and gender from relevance analysis, even identical twins could be recognized thanks to strategic analysis.
2006: The Netflix Award
Netflix created this award which consisted of participants having to create Machine Learning algorithms with the highest efficiency in recommending content and predicting user ratings for movies, series and documentaries.
The winner would receive one million dollars if they could improve the organization's recommendation algorithm, called Cinematch, by 10%.
2009: Fei-Fei Li created ImageNet
Fei-Fei invented ImageNet, which enabled major advances in Deep Learning and image recognition, with a database of 140 million images.
It now consists of a quintessential dataset for evaluating image classification, localization and recognition algorithms.
ImageNet has now created its own competition, ILSVRC, designed to foster the development and benchmarking of state-of-the-art algorithms.
2010: Kaggle, the community for data scientists
Kaggle is a platform created by Anthony Goldbloom and Ben Hamner. It is a subsidiary of Google and brings together the world's largest Data Science and Machine Learning community.
This platform has more than 540 thousand active members in 194 countries where users can find important resources and tools to carry out Data Science projects.
2011: IBM and its Watson system
Watson is a system based on Artificial Intelligence that answers questions formulated in natural language, developed by IBM.
This tool has a database built from numerous sources such as encyclopedias, articles, dictionaries, literary works and more, and also consults external sources to increase its response capacity.
This system beat champions Rutter and Jennings on the TV show Jeopardy!
2014: Facebook develops Deep Face
In 2014, Facebook developed a software algorithm that recognizes individuals in photos on the same level as humans do called Deep Face.
This tool allowed Facebook to identify with 97.25% accuracy the people appearing in each image, almost matching the functionality of the human eye.
The social network decided to activate face recognition as a way to speed up and facilitate the tagging of friends in the photos uploaded by its users.
The History of Deep Learning
MOST IMPORTANT MOMENTS IN THE HISTORY OF DEEP LEARNING
1943: Pitts and McCulloch's neural network
University of Illinois neurophysiologist Warren McCulloch and cognitive psychologist Walter Pitts published "A Logical Calculus of the ideas Imminent in Nervous Activity" in 1943, describing the "McCulloch - Pitts" neuron, the first mathematical model of a neural network.
Their work helped to describe the cerebellum's functions and demonstrate the computational power connected elements in a neural network could have. This laid the theoretical foundation for the artificial neural networks used today.
1960: Henry J. Kelley invents the Backward Propagation Model
In his paper "Gradient Theory of Optimal Flight Paths", Henry J. Kelley shows the first version of a continuous Backward Propagation Model. It is the essence of neural network training, with which Deep Learning models can be refined.
This model can adjust the weights of a neural network based on the error rate obtained from previous attempts.
1979: Fukushimma designs first convolutional neural networks with Neocognitron
In 1979, Kunihiko Fukushima first designed convolutional neural networks with multiple layers, developing an artificial neural network called Neocognitron.
This design allowed the computer to recognize visual patterns. It also allowed the computer to increase the weight of certain connections on the most important features. Many of his concepts are still in use today.
1982: The Hopefield Network is invented
John Hopefield creates the first recurrent neural network, which he calls Hopefield network. The main innovation of this network is its memory system that will help various RNR models in the modern era of Deep Learning.
Hopefield sought to have his artificial neural network store and remember information like the human brain. From pattern recognition, it can detect errors in the information and even recognize when the information does not correspond to what it seeks to achieve.
1986: Parallel distributed processing is created
In 1986, Rumelhart, Hinton, and McClelland popularized this concept, thanks to the successful implementation of the backward propagation model in a neural network.
This was a major step forward in Deep Learning as it allowed the training of more complex neural networks, which was one of the biggest obstacles in this area. The three fundamental principles of distributed parallel processing are the distribution of information representation, memory and knowledge are stored within the connections between neurons, and learning occurs as the strength of the relationship changes through experience.
1989: Yann LeCun demonstrates the backward propagation model in a practical way
One of the greatest exponents of Deep Learning, Yann LeCun used convolutional neural networks and backpropagation to teach a machine how to read handwritten digits.
He showed his work and the results of his work at Bell Labs, the first time such a model had been demonstrated in front of an audience. Watch here.
1997: IBM's Deep Blue beats Garry Kasparov
Garry Kasparov, world chess champion, was defeated by the IBM-built supercomputer Deep Blue on May 11, 1997.
Deep Blue won two games, drew three and lost one, making it the first virtual world chess champion.
1999: Nvidia creates the first GPU
Geforce 256 was the first GPU in history, created in 1999 by Nvidia. This technology would unlock possibilities that were previously only possible in theory. Thanks to this technological component, many advances in Deep Learning and Artificial Intelligence were realized.
2006: Deep belief networks are created
In their paper "A fast learning algorithm for deep belief nets" Geoffrey Hinton, Ruslan Salakhutdinov, Osindero, and Teh demonstrated the creation of a new neural network called the Deep Belief Network. This type of neural network made the training process with large amounts of data easier.
2008: GPU for Deep Learning
10 years after the first GPU was created by Nvidia, the group of. Andrew NG's group at Stanford began to promote the use of specialized GPUs for Deep Learning. This would allow them to train neuronal networks faster and more efficiently.
2012: Google's cat experiment
Google showed over 10 million random YouTube videos to a brain. After being shown over 20,000 different objects, it began recognizing cats' images using Deep Learning algorithms without being told the cat's properties or characteristics. This opened a new door in Machine Learning and Deep Learning since it proved that images did not need to be labeled for a model to recognize the information presented.
2014: Adversarial generative neural networks are created
Ian Goodfellow creates generative adversarial neural networks which opens a new door in technological advances within areas as different as the arts and sciences, thanks to their ability to synthesize real data.
It works because two neural networks compete against each other in a game and through this technique, can learn to generate new data with the same statistics as the training set.
2016: AlphaGo beats the world Go champion
Deepmind's deep reinforcement learning model beats the human champion in the complex game of Go. The game is far more complex than chess, so these feat capture everyone's imagination and take the promise of deep learning to a new level.
2020: AlphaFold 2020
AlphaFold is an AI system developed by DeepMind that predicts the 3D structure of a protein from its amino acid sequence. It regularly achieves accuracy that rivals that of experiments.
DeepMind and the EMBL European Bioinformatics Institute (EMBL-EBI) have partnered to create the AlphaFold database and make these predictions available to the scientific community.
2022: IMAGEN and Dall-E mini
This year, Google and Deepmind launch their two models that can create original images from lines of text fed by users.
This is one of the most important steps within the AI industry, as it allows for the first time to demonstrate the creative capacity of these technologies and the frontiers we could reach when humans work collaboratively with machines.
If you want to learn more about Autonomous Artificial Intelligence, don't forget to visit our complete guide here.
And if you are interested in learning more about Machine Learning and its impact, you can read our article on the topic here and learn about Deep Learning here.