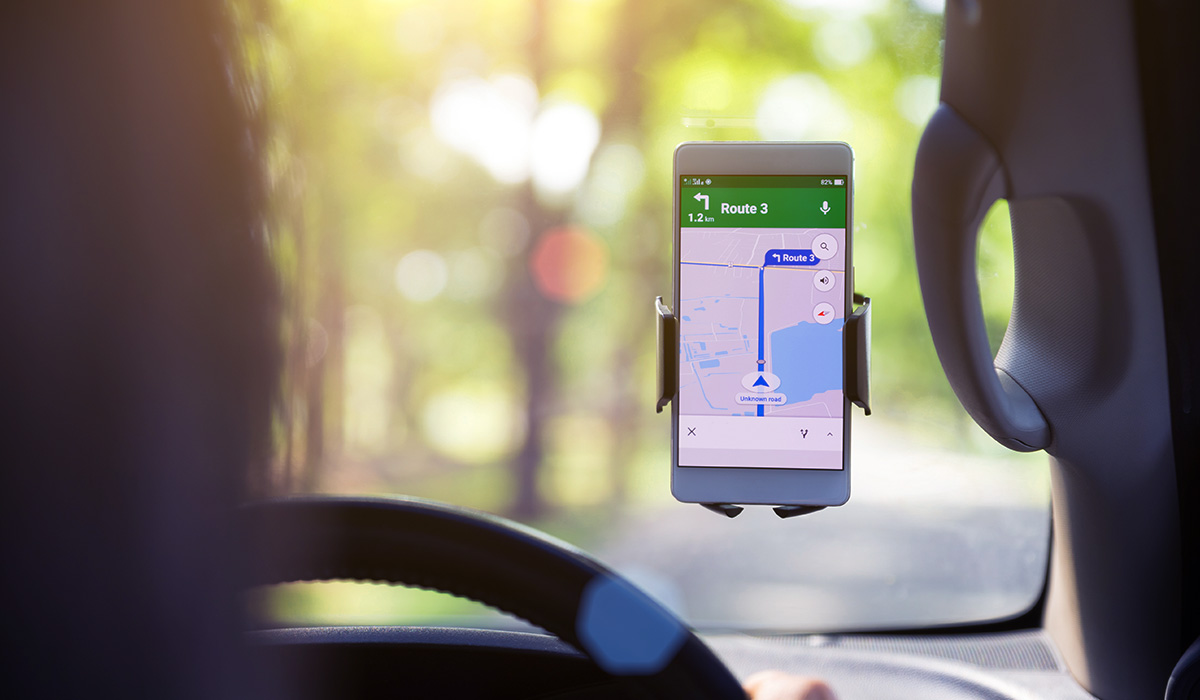
Several Machine Learning examples are already part of our daily lives. And in the coming years, our relationship with this subset of AI and autonomous AI will grow even more. That’s why it’s so important to identify current Machine Learning technologies and the ones that will come soon.
In the following article, we will explore 5 Machine Learning examples that you surely use at least a couple of times per week.
Table of contents
- How does Machine Learning work in today's world?
- 5 most used Machine Learning applications in companies
- Machine Learning Example #1: Google Maps
- Machine Learning Example #2: Uber
- Machine Learning Example #3: Photos iOS
- Machine Learning Example #4: Facebook Ads
- Machine Learning Example #5: Spotify
- Machine Learning Example #6: Amazon
- Machine Learning Example #7: Instagram
- Machine Learning Example #8: Paypal
- Machine Learning Example #9: Netflix
- Machine Learning Example #10: Duolingo
- How does Algotive use Machine Learning?
How does Machine Learning work in today's world?
In a world where more and more information is being created and stored, Machine Learning has become crucial. Today's computational advances have allowed us to create a sea of data, which allows us to understand our surroundings better and the ways we interact with our environment.
However, it is humanly impossible to comprehensively review all this information and derive valuable insights from it. This is where Machine Learning comes into play.
Machine Learning has managed to use this outpour of information effectively. When a machine is "trained" to perform a task through its experiences with large datasets, the machine can later perform the assigned task by itself automatically. Moreover, this technology can predict future outcomes and optimize processes to achieve higher performance.
Learn more about what Machine Learning is and how it works in our article here.
5 most used Machine Learning applications in companies
Machine Learning has become an invaluable ally for organizations due to its great capacity to organize, predict and reduce complex processes in favor of efficiency.
Companies find significant advantages in using this technology in their daily operations to automate several tasks and improve their performance across different areas.
“83% of organizations have increased their Machine Learning budgets year over year”- Algorithmia
These are the 5 most common applications in companies using Machine Learning:
1. Predictive Analytics
This Machine Learning application can predict what will happen based on historical data and can identify patterns from this information. Companies use this Machine Learning application to eliminate risks, reduce costs and identify areas of opportunity for the business and its employees.
2. Knowledge extraction
This Machine Learning application allows the processing of large amounts of structured and unstructured data to identify covert relationships. By tracking recurring information, this ML application can transform millions of pieces of data into valuable insights.
3. Image recognition
This Machine Learning application defines a set of objects to be identified in images by training a model with labeled photos. This helps companies in security and productivity matters. Deep Learning has boosted this application making it even more powerful.
4. Behavioral analysis
This Machine learning application builds baselines of normal behavior for each user in specific scenarios by observing historical activity and comparisons within peer groups. In this way, the behavior and reactions of specific individuals in various scenarios can be identified.
5. Recommender systems
Recommender systems are concerned with ranking products and user groups. Generally, a recommender system predicts the ratings that a user might give to a specific item or content. These predictions are ranked and returned to the user with similar results they might be interested in.
5 APPLICATIONS OF MACHINE LEARNING IN BUSINESS
Machine Learning Example #1
Google Maps is one of the most famous applications of this company. But the capabilities of Google Maps were not even possible until a few years ago. This application uses two main factors in its favor that no other organization has: a large amount of information from its users and Google's development of their Machine Learning platforms with cutting-edge technology.
How does Google use Machine Learning?
To accurately predict future traffic, Google Maps uses Machine Learning to combine live traffic conditions with historical traffic patterns on roads around the world. This allows it to show specific information to its users about the estimated time of a route and the real-time traffic to get there.
Machine Learning Example #2
Machine Learning is the backbone of Uber. Since they started operations, this American company has been keen on using artificial intelligence to make the user experience better and unique.
But without constant innovation, this would not be possible. Uber has bet on Machine Learning like a no other organization to stay at the top.
How does Uber use Machine Learning?
Uber developed its own Machine Learning platform called Michelangelo. In it, its developers can create Machine Learning models to power the company's applications. One such development was a Customer Obsession Ticket Assistant (COTA) tool to help agents provide better customer service.
COTA helps resolve up to 90% of incoming support tickets quickly and efficiently.
Machine Learning Example #3
The way we store our photos has changed tremendously due to Machine Learning. Now we can take a photo at any time and place, and also we can categorize them in our digital library, or create collages automatically and identify particular features thanks to this technology on our mobile devices.
How does Apple use Machine Learning?
Apple uses a series of Machine Learning algorithms in its Photos app for iOS that run privately to help curate and organize images on users' iPhones. This app further learns from a user's interesting patterns and identifies everything from groups of important people, frequent locations, past trips, and events, etc.
Machine Learning Example #4
Marketing is one of the industries that has been transformed in the last decade thanks to Machine Learning.
The tools that Meta (formerly Facebook) has developed for businesses on its social media platforms in recent years have revolutionized digital advertising campaigns. From measuring the results of a campaign in real-time to recommending audience segments that could be interested in specific products or services, this company changed Internet advertising thanks to Machine Learning. However, they have been criticized for their privacy policies in recent years.
How does Meta use Machine Learning?
With the information that Meta has on its platform, the advertising efforts of its advertisers achieve a more substantial impact due to Machine Learning. As more people view an ad, share comments about it or click to purchase on an advertiser's website, Meta's Machine Learning models improve the prediction of estimated action rate and ad quality. This maximizes value for individuals and businesses.
Machine Learning Example #5
One of the most popular cases of Machine Learning in the music industry is Spotify.
With more than 406 million users today, this company's relationship with artificial intelligence is unprecedented. Thanks to its vast music catalog, user-created playlists, and millions of behavioral data from its subscribers, this company has been able to leverage the immense amounts of information at hand in successful and, above all, creative ways using Machine Learning.
How does Spotify use Machine Learning?
Spotify uses Machine Learning for its music recommendations in three main ways.
- Collaborative filtering: Based on data from its user base, Spotify groups different listeners based on their specific tastes and recommends content similar to what they regularly enjoy.
- NLP: Based on neurolinguistic programming, information can be identified with tags within each song or playlist that users create. In this way, Spotify can better understand why people are interested in certain songs or genres.
- Audio modeling: This type of ML algorithm explores the song's content and compares it with other songs to identify categories. For example, if a song has a particular rhythm or certain instrumentalization, it will be categorized within playlists of similar genres and tunes.
Machine Learning Example #6
While Amazon is known mostly for its efficient system of deliveries and its smart suggestion of products, it is also gaining strength as a technology and software developer. In the last few years, they have launched several applications and even video games. However, their most popular software is the smart assistant, Alexa. This software, similar to Google Assistant, Apple’s Siri, or Microsoft’s Cortana, has managed to enter millions of houses all around the world providing services like organizing calendars, setting alarms, telling jokes, and even holding small conversations from programmed scripts.
How does Amazon’s Alexa use Machine Learning?
Smart assistants can learn new skills that can be accessed by the user through voice. In the US, there are more than 70,000 skills available, and every day new features arrive that add value and information to Alexa’s large database. Alexa uses knowledge extraction to recommend songs, predict the weather, or recommend products from Amazon’s website. It is also able to read aloud emails and e-books, and since it has no visual interface, it has proven to be of help for visually or physically challenged people. The user can also make notes and reminders that can be read as text in the accompanying app.
Machine Learning Example #7
While Instagram is now part of Meta, it started as its own simple thing: an application for taking photographs, adding filters to them, and sharing them with your friends. However, it has become more and more complex over the years, as new features are added that use Machine Learning to enhance the user’s experience. Nowadays, there are more than 2 billion users on this popular social network, and all of them have been using Machine Learning, most of them without knowing.
How does Instagram use Machine Learning?
Instagram uses Machine Learning in several ways. For example, it uses behavioral analysis to offer tailor-made content for each user. The platform gathers information such as liked photographs or visited pages to offer the user similar content available within the millions of posted pictures or videos.
It also considers the things that are of no interest to the user, like blocked users, words, or other content that is simply ignored. This has become more evident with the implementation of Reels, a video-based new feature of the platform: when entering the Reels section, Instagram’s algorithm learns the patterns of behavior of the user within that platform and suggests content suited to the user. In the creation of these, as well as of regular photos, facial recognition has proven pivotal, as the software recognizes the face and its movements to apply particular effects.
Machine Learning Example #8
As online transactions become more and more common around the world, it must not come as a surprise that sites like Paypal are part of our everyday lives whether we want it or not. The site, originally founded in 1998, has reached the 200 million users milestone. Paypal’s clients constantly use the platform’s online transactions to avoid queues at the bank and the risks of carrying around large amounts of money. As a business that deals with other people’s money, Paypal must ensure the safety of their site and their customers’ values, and some of their strategies to achieve this is the implementation of Machine Learning in their security systems.
How does Paypal use Machine Learning?
Since there are millions of transactions every day through Paypal’s website, it would be impossible for the human eye to monitor each of these and detect fraudulent transactions. Instead, Paypal implements algorithms to scan large amounts of data for the sake of financial accuracy; this means that it extrapolates patterns and pattern deviations from user activity, such as location, deposits, transfers, or other useful data. Paypal’s website can assess customer behavior in real-time and flag situations in which a legitimate user starts behaving erratically within the platform. Through machine learning, Paypal has been able to adapt to the new patterns of people that commit fraud, remaining one step ahead of them by flagging certain domains, devices, IP addresses, and common behaviors used by these fraudsters.
Some of the most common types of fraud on this website are Signup fraud (creation of fake accounts), Login fraud (hijacking of an existing account), and Payment fraud (the use of money without the real user’s knowledge) (https://www.paypal.com/us/brc/article/payment-fraud-detection-machine-learning).
Through Machine Learning, Paypal has managed to automatize the detection of these types of fraud, reducing them significantly and ensuring that the money of its users is as safe as possible.
Machine Learning Example #9
Nowadays there are plenty of platforms that offer video streaming, but one cannot deny the importance of Netflix as one of the pioneers. With more than 160 million members in more than 190 countries, this famous streaming platform owes its popularity not only to the quality of its original series, feature films, or documentaries but also to its recommendation system, which relies (of course) on Machine Learning.
How does Netflix use Machine Learning?
Netflix’s algorithm uses predictive analytics to customize the content it will recommend to every user. Using data mining, statistics, and modeling, the platform analyzes the patterns of content-watching of the users and goes deep into its database to search for users with similar tastes and viewing habits. Based on this, Netflix can predict what kind of content someone will like, and said content will appear on the home screen of that user. This ensures that the customers always have something to watch akin to their tastes.
Machine Learning Example #10
Duolingo has become one of the most popular platforms for learning languages. Founded in 2011, the app has more than 56 million users active each month and can be accessed on any smartphone or computer that has an internet connection. While this seems to be a simple program with no need for complex Machine Learning solutions, one would be surprised as to how the application known for its green owl mascot named Duo implements AI on its platform.
How does Duolingo use Machine Learning?
Perhaps the most obvious instance of Machine Learning is the speech recognition feature of the platform in which the user’s pronunciation, accent, and use of spoken language are evaluated against a large database of linguistic samples that expands with every entry. But a more complex instance of Machine Learning is also at work within the “Report” feature of the platform.
Users can report any errors in the exercises or interface of the platform by using a “Report” flag button that appears after every answer. However, as might be expected, a lot of reports are sent each day and there are too many to be checked by human means. Thus, Duolingo has implemented a logistic regression system to detect, within the myriad of reports, which are worthy of human review, which have an easy automated fix, and which can be ignored.
This system considers the correct grammar of the language, the user’s usual performance, and the data within the application’s code to determine the relevance of a report. This makes the developer’s job easier and helps them offer the best product to the many users that just want to learn a new language. (https://blog.duolingo.com/how-machine-learning-helps-duolingo-prioritize-course-improvements/)
How does Algotive use Machine Learning?
At Algotive, we take Machine Learning capabilities to a new level, thanks to our autonomous artificial intelligence.
People can make critical decisions with an unparalleled technological ally with our video analytics applications. Algotive's technology performs highly critical tasks, without major human intervention, with a high degree of accuracy and effectiveness.
Autonomous AI is the next logical step in our relationship with technology. Read our complete guide here if you want to learn more about our autonomous artificial intelligence.